Artificial Intelligence – The Good, The Bad, and The Ugly
In 2018, you just can’t hide from the media frenzy around Artificial Intelligence. Statements like “CIOs must put AI on the fast track”, “the local business sector is well behind foreign competitors”, “totally unprepared”, “we have to catch up”, and “Elon Musk and tech heavies invest $1 billion in Artificial Intelligence” dominate the headlines. At the same time, just about every software application with semi-advanced algorithmic capabilities now claims to include AI.
When I received an invitation to talk about AI at the Online Retailer conference in July 2018, under the heading of ‘The Good, The Bad and the Ugly’, I decided to focus on two topics. Firstly, I felt it necessary to clarify what the term ‘AI’ really stands for, to enable a better understanding of AI’s potential and pitfalls. Then I could paint a picture of how this technology will impact businesses, particularly retailers, over the next few years.
I was honoured by the volume of positive feedback received after my keynote, which indicated that my mission was a success. Given the number of requests I have received for the information I shared, I thought it would be of value to summarise my presentation into an article.
During the keynote, given the media buzz around AI, I started by pointing out that often a popular opinion can be misguided, if not totally incorrect. I displayed advertising posters from the 1950s, promoting cigarettes. At the time, both Lucky Strike and Camel cigarettes had endorsement from over one hundred thousand doctors and medical practitioners in the US. Could the same irony be true today for those who promote AI as not only a silver bullet for retail success, but also as essential for mere survival?
You may question my skepticism, witnessing AI-enabled machines performing ever more impressive feats. I showed the audience two short videos to highlight the point, featuring dog-like robots collaborating to open and walk through a door, and a hard-working industrial robot deburring a complex metal structure.
One interesting detail I added later: the dogs were from 2015, but the robot dates back to the 1970s. If the dogs can be considered Artificially Intelligent, then surely so can the (old) robot. I suggested to the audience that perhaps a well-established technology has being repackaged and sold to us as a recent breakthrough. The primary selling technique seems to be feeding on our fear of missing out.
As you can imagine, I set an interesting scene to explore.
AI – what does it actually mean?
In response to this rhetorical question, most people would say: ‘Artificial Intelligence’ – a universally accepted, yet incorrect answer.
I showed the audience the definitions of the component words in the misinterpreted AI acronym:
- Artificial: being the product of intentional human manufacture, rather than occurring naturally. No problems here.
- Intelligence: capacity for logic, understanding, self-awareness, learning, emotional knowledge, reasoning, planning, creativity, and problem-solving. Here lies the glitch: self-awareness? Can anyone intoxicated with the ‘promise of Artificial Intelligence’ demonstrate even a sliver of self-awareness in their machines? I doubt it.
In the seemingly intelligent contraptions I showed in my short videos, Artificial holds true, however, self-awareness, emotional knowledge and creativity do not. Even our most advanced understanding of the human brain fails to explain our consciousness. How can we replicate (using computers) something we do not understand?
The following quote from the Harvard Business Review illustrates well the abuse of the term of ‘intelligence’ in the AI field: “Roger Schank, a researcher and former professor, once proposed a novel goal for artificial intelligence: A computer should be able to watch West Side Story and recognise the plot of Romeo and Juliet. Schank and his students believed that stories are central to intelligence, reasoning, and meaning. By Schank’s measure, today’s AI isn’t intelligent at all.”
So, if the ‘I’ part in AI does not qualify as Intelligence then what does it really stand for? We live in a world swarmed over by increasingly smart machines. They listen and talk to us. How can we accurately describe this advanced technology?
First, let’s cross out some words from the definition of intelligence: capacity for logic, understanding, self-awareness, learning, emotional knowledge, reasoning, planning, creativity, and problem-solving.
Next, we just need to add pattern-based planning and a ‘new’, well-known phenomenon emerges: Instinct.
Welcome to the world of Artificial Instinct, which gives the Appearance of Intelligence. I don’t mind using any of these two interpretations of AI: both reflect the true nature of the technology in question.
Artificial Instinct at work
As redefined, AI (Artificial Instinct that appears to be intelligent) has been around for many years, but has recently started to gain material inertia due to steady advances in the underlying technologies.
Just like the robot dogs and the robotic machine arm in my opening videos, you can now witness Artificial Instinct at work in many areas of life – car navigation systems, smartphone screens, data search engines, image analysers, X-ray/MRI interpretations machines (now more accurate than humans in cancer detection), washing machines, house-wide climate control, video games, board games, Siri/Google Home, and Alexa.
I highlighted Alexa, because I used her to jokingly illustrate that we still have a considerable distance to travel to get such systems to a reasonable level of reliability.
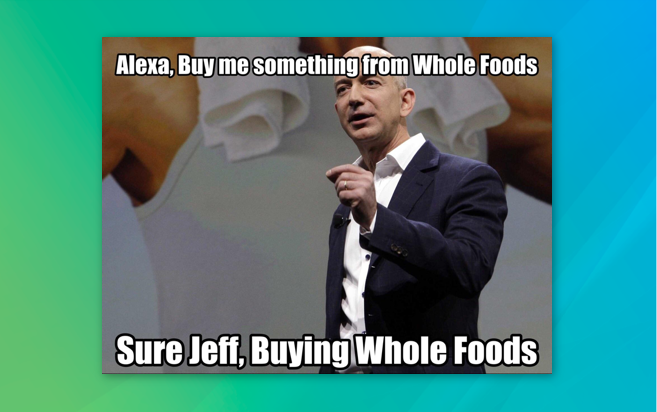
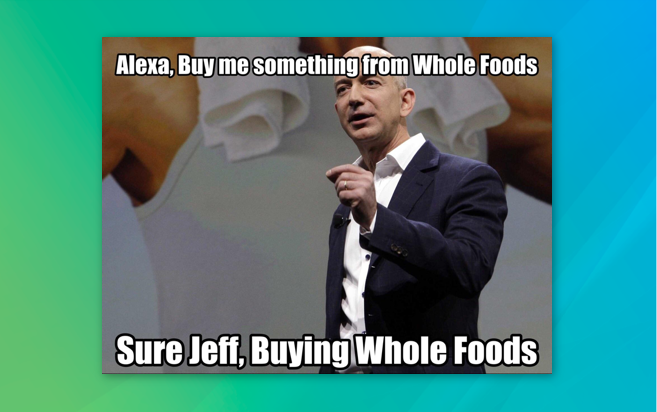
Recent statistics show that only 2% of the 50 million people who have purchased Alexa devices used them for shopping. Furthermore, of those who did, 90% never used it a second time to place an order.
This confirms that though Artificial Instinct tech keeps thriving around us, we are not even close to replicating intelligence. It would be commendable if the technology industry could admit to this and, consequently, the media would tone down their overhyped Artificial Intelligence narrative.
Another example: the OpenWorm project has been active since 2011 aiming to replicate the nervous system of a 1mm non-parasitic worm digitally. Seven years on and the project can only be considered 20-30% complete – at best.
What does this tell us about our ability to create true, human-like Artificial Intelligence? I have no doubt that the achievement of General Artificial Intelligence won’t happen for the next 30 years, if at all. If our consciousness resides even partially outside the physical brain, we most likely will never be able to replicate it in a computer. The mechanical, hydraulic and chemical features of our brains can’t be replaced with wires either.
Neural Networks and Machine Learning
After having explored the true premise behind Artificial Instinct, I embarked on a rudimentary explanation of how it actually works. It was time to talk about neural networks and deep learning.
I talked to a commonly used high-level diagram, which illustrates a neural network system.
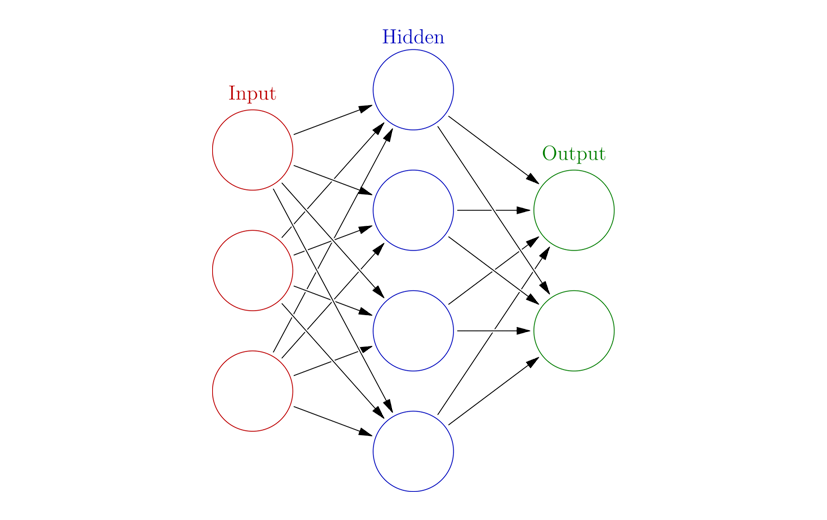
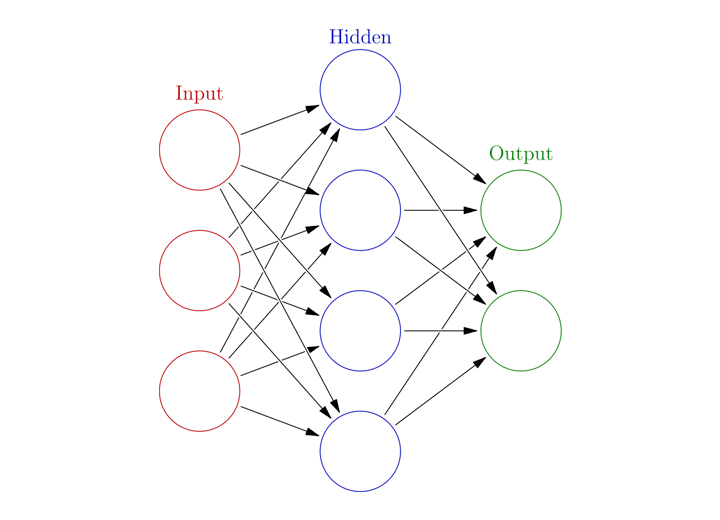
The red circles take inputs from the outside world via e.g. cameras, microphones, a database, or from any other sensors. Obviously, a single system can have millions of such red circles.
The inputs, once normalised (converted to relative numbers), flow on to the blue circles where they undergo transformation. The ‘smarts’ can be found in the transfers from the red to blue circles (represented by black arrows) and within the blue circles themselves.
During the transfers, the system multiplies the inputs using weights (to amplify or dampen them) and within the blue nodes the system uses the weighted inputs as parameters for functions, typically non-linear. Such functions describe the behaviour of dynamic, real-life systems. The output of the computations flows into the green circles, which could generate an image (or a fast sequence of them, giving an illusion of motion), a printout or synthetised voice.
If you repetitively expose such a system to e.g. pictures and provide feedback whether the output reflects what you want the system to ‘see’, it will learn. The ‘learning’ occurs because in each try the system adjusts the weights between the red and blue circles, as well as the parameters of the functions within the blue circles. Very smart blue circles will even switch to another function – all aimed at giving the ‘trainer’ the answer the trainer expects. Note that the system could also be programmed to take notice of input frequencies and in the process recognise statistical patterns, to be used in generating subsequent answers.
If such a system has more than one layer of the blue circles, the experts will say that it has the capability for ‘deep learning’. Our example had only a single blue layer.
Clearly, we won’t find any intelligence here. Just voice, image or other data capture, millions of calculations in-between (using formulas augmented by previous calculations), and then the generation of voice, image or just data.
What causes all the AI noise in the media?
As visible in our daily lives, smart machines relentlessly proliferate, getting more advanced at an exponential rate. Artificial Instinct has made machines incredibly useful, with many profound applications across all industries.
Therefore, the media can be justified in their enthusiasm, but it doesn’t do anybody any good by portraying AI as Intelligence or something close to it. With a fundamentally flawed understanding of this rapidly evolving technology, people have made (and will continue to make) mistakes: technical, financial and social.
One of the key culprits in the confusion stems from today’s hardware giving the Appearance of Intelligence – machines can talk and respond to voice. Add to this the explosion of mobile hardware and you get moving machines with the ability to interact verbally with people. This can spook and fool anyone.
The construction of these machines has become possible due to massive increases in data storage, the power of the CPUs, battery life, and networking – with lower and continually declining costs for all of the above.
Another driver of the AI-related media noise comes from AI’s quite frightening potential for military use and automated cyber-crime. This dark side of AI extends to people having concerns about even normal AI machines causing them harm – intended or unintended.
Related to the above, the unclear regulatory framework caused by the confused understanding of AI has led to a somewhat irrational push from various directions for governments to impose limits on AI research and developments. Every ethical angle has been debated in an attempt to figure out who ought to be held accountable when an AI machine goes rogue and hurts or kills people.
I offered a simple suggestion to cut this debate short: if we dispense with the notion of ‘Intelligence’ and start talking about Instinct, then if dog owners must be responsible for their dogs’ behaviour, then why not for that of their machines? If you don’t know what your contraption will do, don’t put it out into the world (particularly on the road). The proponents of self-driving cars should think twice – most cities can’t even get their trains to run automatically, on stringently controlled tracks. What chance does a car have, in a thousand times more complex environment?
In trying to explain the media hype, one also can’t ignore the power of marketing – companies and people with vested interests want to sell the ‘next big thing’. No wonder they have jumped on the media bandwagon in an attempt to cash in on the hype.
Artificial Instinct in retail
Armed with the competitive advantage of understanding AI as Artificial Instinct, we can now look at its applications and potential in retail.
Let us start by going back to our simplistic AI machine: it relied on red circles accepting and normalising inputs from the world – via sensors or from a database. This tells us that before a smart system can be deployed and ‘educated’, basic data management must be in good shape.
If the input data lacks completeness, timeliness, coherence and accuracy, the smartest machine learning in the world will produce an “educated imbecile”. Think of the damage this could cause in your business.
This means that the key prerequisite for bringing AI into retail must be the creation of a single source of all retail information, with properly structured and timely data, to reflect the real world. This basic objective remains a real challenge for most retailers.
Only when your core data begins to look solid can analysis tools be deployed to interpret and use the data (note: we haven’t yet touched any AI technology). Retailers can realise massive benefits just from implementing Information Management 101. Simply maintain good data, and then start analysing and acting on it. The more such data gets used, the better it will become, as users will object to detected inaccuracies.
Once your organisation develops a data hygiene and utilisation culture, you can embark on bringing Artificial Instinct into the enterprise. But, start small with Primitive AI. For example, a retail chain with 1,000 stores and 100,000 SKUs must make 100 million decisions to replenish the stores. This begs to be automated before anything else. Start by putting in place smart algorithms: to forecast demand, to identify best sources of stock, estimate customer traffic, transport requirements, shipping times, etc.
Once all the basics have been mastered, then you can bring in learning platforms, which can automate more complicated human tasks, take actions that humans would not be able to decide upon, and evolve their algorithms as well as efficiency along the way.
Without such a sober process, Garbage-In-Garbage-Out will occur, just with more smarts than in 1960s when the term was coined. I wish I had less imposing guidance for you. Unfortunately, actualising the true benefits of AI requires an understanding of the vital importance of the architecture in retail systems management, an acute strategic focus, and a high level of organisational discipline. Few retailers can boast all of this.
Conclusion – the path forward
While Artificial Instinct has a lot of promise for retail operations, introducing it into your business requires a structured approach:
- Don’t be afraid of missing out, invest time to get the basics right first
- Just getting the basics right will return material benefits
- Don’t forget to be a good retailer in the meantime; good stock, at a right price, in the right place will always make you money
- Focus on mastering the Digital Path to Purchase and to get there merge your e-commerce and marketing departments
- Bring in AI but just as another tool, don’t expect it to solve all your problems
I recall in the animated movie Kung Fu Panda, all the warriors wanted to learn the secret of the Dragon Scroll, which contained the key to “limitless power”. However, when the scroll was finally opened it was found to be blank, showing only the reflection of the reader.
The lesson: in retail too, it would be a mistake to search for a secret ingredient. The power to succeed rests in you.